The 2020, 2021, and 2022 MLB pennant race charts using Retrosheet data have been updated on the Exploratory Server: https://exploratory.io/dashboard/kc2519/Pennant-Races-1901-current-aUu5vDT1EW. All seasons from 1901-2022 are now available using the simple parameter selection (just make sure it’s set to the interactive mode).
Here’s a screenshot:
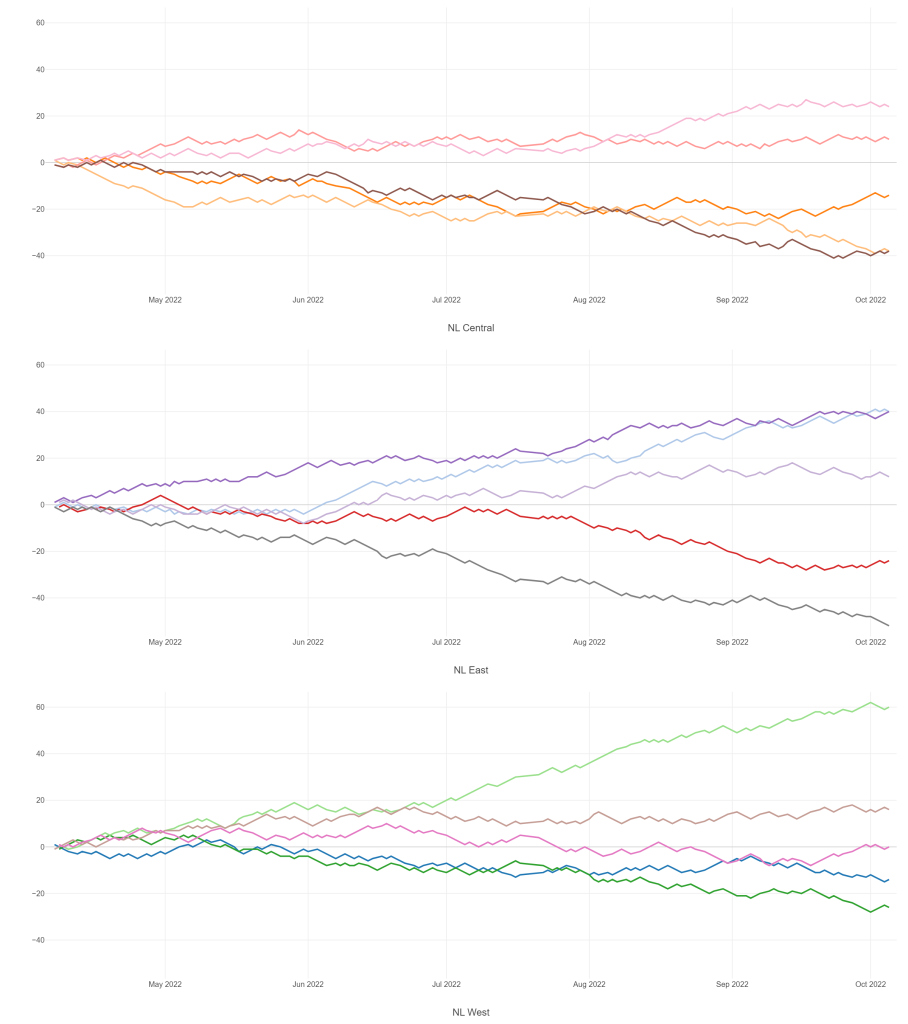
Meanwhile, I’m struggling with some JSON output for my traditional version, so no updates there yet.