One of my ongoing visualization projects has been the Batting Explorer, a semantic-based discovery concept built using the Simile Exhibit open source tools. I’ve been updating this on a not very timely basis the last few years, but have now caught up through the 2015 season. Of course, 2016 stats will be available in the next 2-3 months, so it will be time to repeat the process once more. For the moment, I’ve just added the 2014 & 2015 seasons into one of the decade-based examples, so you can now search for all batters covering seasons from 1901 through 2015.
The explorers work in much the same way as many travel sites you’ve visited on the web. Each page can be filtered using a wide array of facets (filters) that allow you to quickly narrow down results by team, season, batting category, and a bunch of other options. I’ll show this in a moment. Let’s first start with a basic view of the 2010-15 explorer:
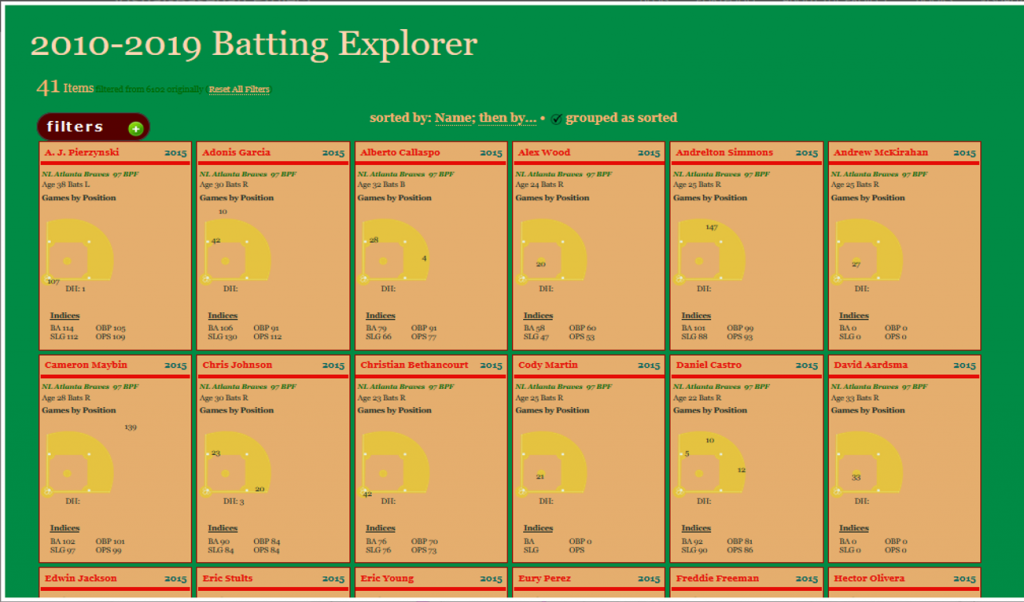
Each of the Batting Explorers has a consistent look & feel, with the underlying data as the only difference. All individual player-season combinations are laid out in a baseball card sort of format, although you can’t flip them over or get any bubble gum either 🙂 Nonetheless, each card contains a wealth of information, including the number of games played by position, laid out on a baseball diamond. In addition, hovering over a card loads a pop-up summary of the season for each individual batter, as seen here:
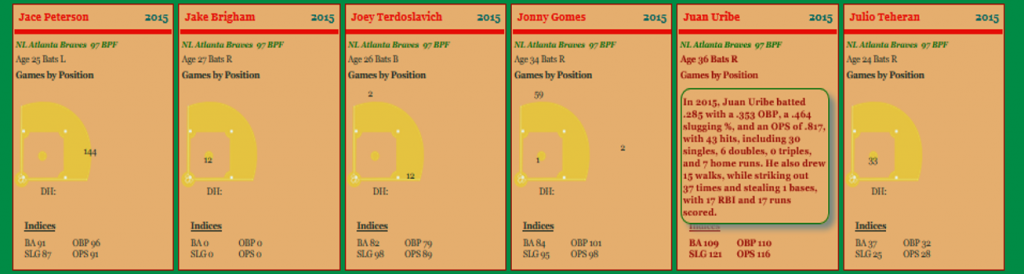
An additional benefit comes when you click on a selected card. Every batter card has a personalized link to the massive Baseball-Reference.com site. Here’s what you’ll see when clicking on the Juan Uribe link:
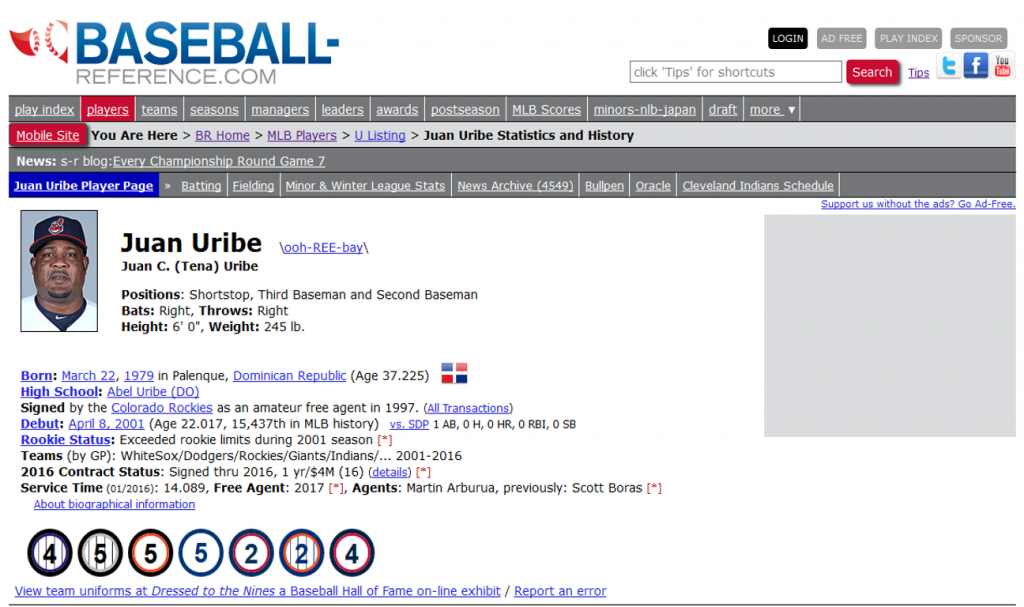
I mentioned earlier the ability to filter using a wide range of facets. Here’s a glimpse of the many categorical and numerical options present in each Batting Explorer:
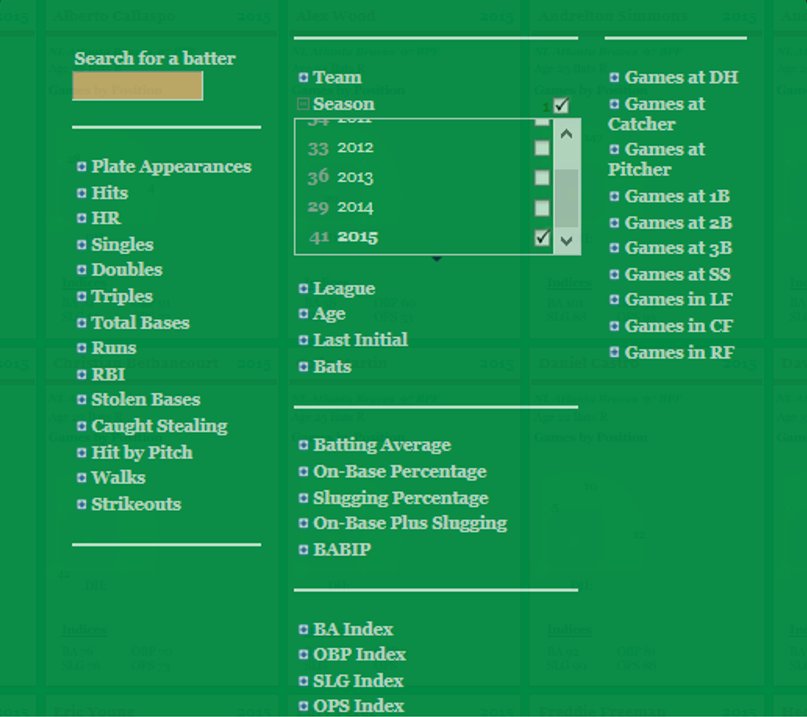
As you can see, there are dozens of possible filters that can be used. If you want to see only batters with more than 40 home runs in a season, simply select the HR facet and check the conveniently provided ranges. Or how about viewing players from a single team? Simple, using the Team facet. Likewise for filtering by season, number of doubles, stolen bases, walks, strikeouts, and so much more. And of course these filters can be used together to quickly find matching results.
Finally, there are a multitude of sort capabilities, or you can choose to have nothing sorted. If you do wish to choose one or more sort attributes, here are your options:
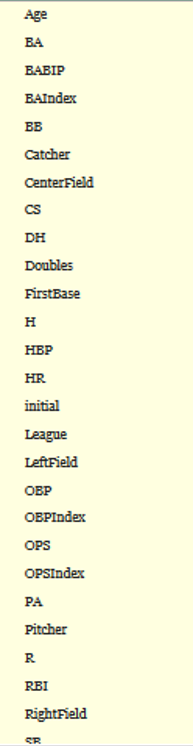
Your sorts can be many layers deep – just keep adding variables!
This has been a very brief overview – to learn more, go to the Portfolio section and begin exploring! While you’re on the site, take some time to view the Game Summary exhibits, set up in much the same fashion using Exhibit. Or, if networks are your thing, check out a large collection of franchise player or team trade networks. Hope you enjoy the site, and thanks for reading.